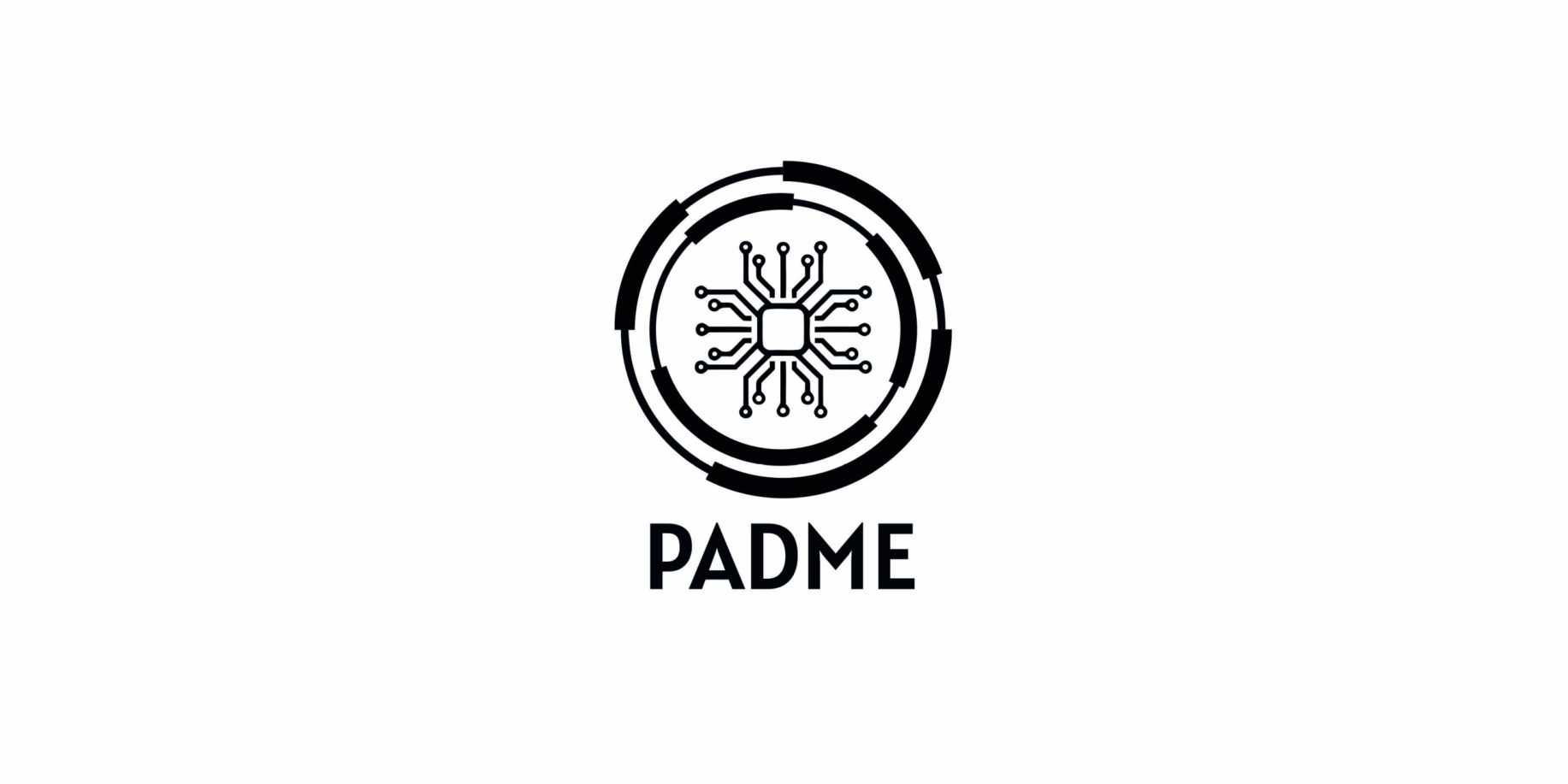
Data stays at the site: PADME platform facilitates privacy-compliant analyses of medical data
Distributed data analysis is of great importance when analyzing large amounts of personal data. The “Platform for Analysis and Distributed Machine Learning for Enterprises” (PADME) established within the SMITH Consortium will facilitate distributed analyses with medical data in the future.
PADME makes use of the Personal Health Train concept (PHT). The PHT pursues a distributed data analysis. Unlike previous approaches, the analysis comes to the data instead of all relevant data first being centralized and then analyzed. This allows potentially sensitive data to remain protected at its location.
The approach of the Personal Health Train is comparable to transporting goods by rail. Data analyses are carried out at the data-holding institutions one after the other. The institutions represent different stations that the “Data Analysis Train” travels to.
Privacy-protected analyses with the Personal Health Train
Through the PADME platform, data-holding institutions can register to contribute selected medical data to the analysis of a research question. To do this, researchers provide analysis programs; in the best case, they use a program already managed by PADME. These programs are sent from site to site in accordance with the PHT approach to analysis, where they take the results obtained from the data and pass them on to the next site. The data remain at their point of origin and are not viewable by the researchers during the analysis. Only the program itself gains access to the relevant data at any given time. At each site, a separate administrator determines data access.
Following the PHT concept, different health care organizations, hospitals, clinics or other institutions providing health data can take on the role of a station, i.e. a participating partner, in PADME.
A wide range of data analysis options – independent of programming language
The spectrum of potentially possible data analyses ranges from simple descriptive statistics and various types of regression analyses to complex machine learning algorithms that can be used to process different types of medical data (including tables, images, texts, and much more). PADME is not limited to the use of a programming language to implement data analysis. Researchers using the platform can write the analysis programs in the programming language that suits them.
“We urgently need such infrastructures as PADME to address challenging medical questions with modern methods of informatics. Artificial intelligence methods are undoubtedly among them,” says Prof. Dr. Oya Beyan, professor of medical informatics at the University of Cologne and head of the PADME project. PADME has already provided proof of this in various studies and data analyses. One evaluation, for example, focused on the classification of skin lesions in the field of dermatology. This was based on images of the lesions as well as descriptive demographic and anamnestic data on the patients. The data used for this purpose were distributed over three sites and stored in a FHIR server according to the core data set of the Medical Informatics Initiative. In further studies, the functionality and methodological quality of the platform were tested and optimized on this basis.
Sustainable benefits for research and healthcare
In compliance with data protection requirements, the PADME platform can thus be used to incorporate new data sets into medical research. The long-term goal is to improve patient care.
Prof. Dr. Toralf Kirsten, Professor of Medical Data Science at the University of Leipzig and one of the central contributors to the PADME project, emphasizes the importance of the platform for improved research and healthcare: “The PHT concept and the PADME platform are what makes research with large-volume data managed at different institutions possible in the first place. In the future, it must be possible to integrate such highly innovative approaches and products from informatics more strongly into medical research, so that their results can be used to implement patient care even more quickly and in a more specialized manner.”
RWTH Aachen University, Fraunhofer FIT, the University of Cologne, the University of Leipzig and Mittweida University of Applied Sciences have been working together on the PADME platform since 2019. It was initiated with funds from the SMITH Consortium, funded by the Federal Ministry of Education and Research. The further development and adaptation of the project was funded in cooperation with the use cases CORD and POLAR of the Medical Informatics Initiative and the Federal Ministry of Health Consortium LEUKO-Expert. The central services are operated by the Fraunhofer Institute for Applied Information Technology (FIT). “PADME and the Personal Health Train are a paradigm shift for the analysis of data: Protecting data – by transferring analytics to data – is critical not only in healthcare, but also in many other application areas. I am pleased that the PADME team is making an important contribution to this paradigm shift,” explains Prof. Dr. Stefan Decker, Chair of Computer Science at RWTH Aachen University and Director of the Fraunhofer Institute for Applied Information Technology. The current focus of the project is on extending the platform to further improve the usability of the used approach.
How researchers and data-holding sites can use PADME
Researchers can register to use PADME at www.padme-analytics.de to access the analysis programs already created there and more. Data-holding institutions can register via a central registration page. After new registration, the required software is made available.