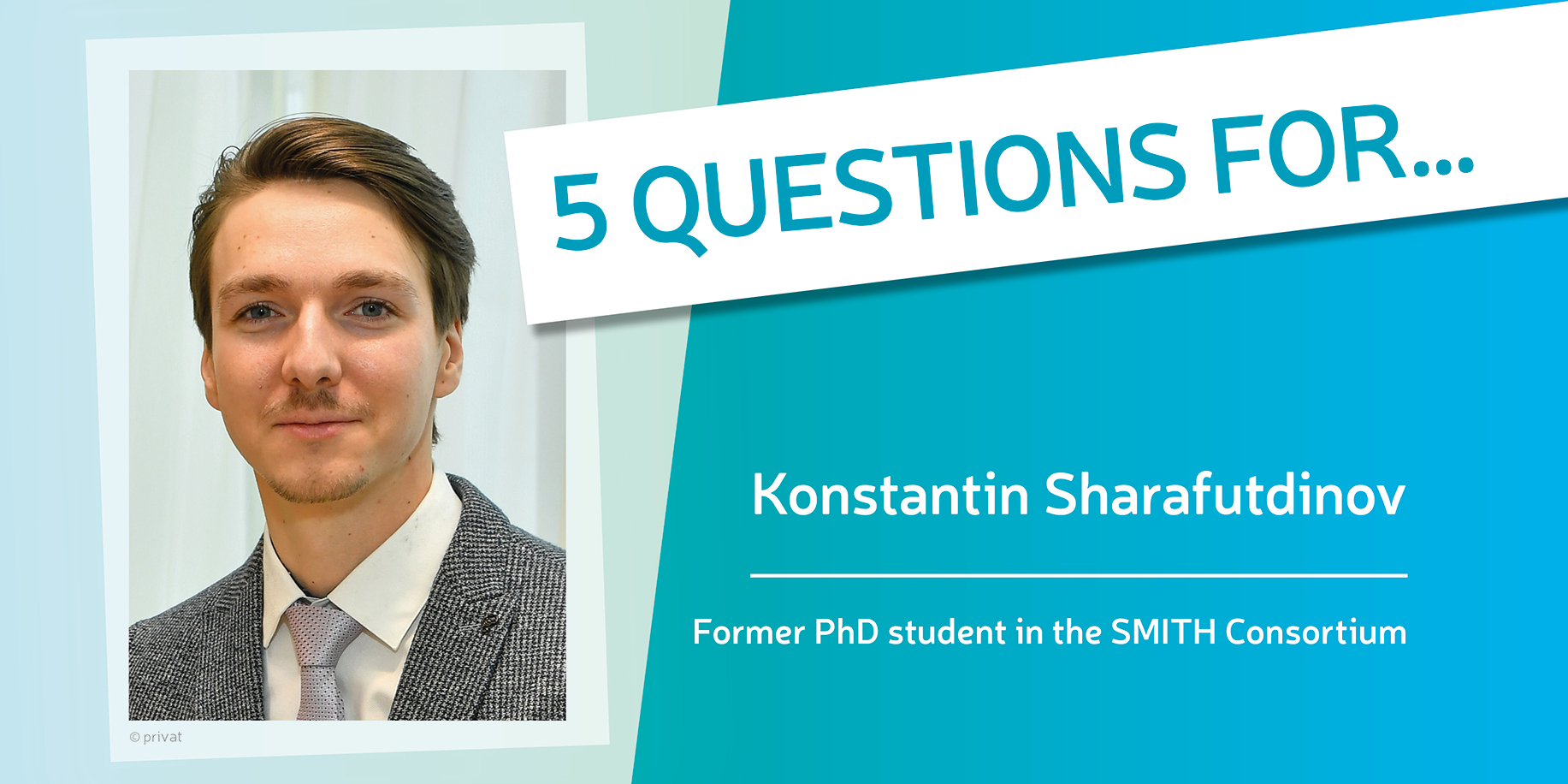
About Virtual Patients and Artificial Intelligence in the Intensive Care Unit | 5 Questions for… Konstantin Sharafutdinov
The current rapid developments in artificial intelligence (AI) and machine learning hold great potential for optimising intensive care medicine. For example, these technologies can be used to predict the course of disease and thus prevent exacerbations. However, there are still some challenges in the practical application of AI in the intensive care unit: Large amounts of data from different sources are needed to train corresponding prediction models. However, since the data structures often differ greatly between hospitals, this can lead to distortions in the prediction. At the University Hospital RWTH Aachen, a research group led by Prof. Dr. Andreas Schuppert, Professor at the Institute for Computational Biomedicine at RWTH Aachen University, has tackled this problem. The research group has modelled Virtual Patients, which can record specific characteristics of patient conditions independently of the hospital. The Virtual Patient Model was tested using acute respiratory distress syndrome (ARDS) data from the ASIC Use Case of the SMITH Consortium. The work of this research group resulted in a paper published in the IEEE Open Journal of Engineering in Medicine and Biology in February 2023. The first author is Konstantin Sharafutdinov, who was a PhD student in the SMITH Consortium from 2018 to February 2023.
In the interview with him, you will learn how the virtual patient can support AI technologies in the intensive care unit and what the future of artificial intelligence in medicine could look like.
You have a Master’s degree in physics and are writing your doctoral thesis on the topic of artificial intelligence (AI). What sparked your interest in this topic?
My interests have always been interdisciplinary. In my Bachelor’s degree, I gained basic knowledge in maths and physics. There I already got a first insight into the world of machine learning. I wrote my bachelor’s thesis in a bioinformatics lab. At that time, we compared genomes of bacteria from patients and healthy people and described the differences. That’s when I first got involved with data-based methods. After my Master’s in biophysics, I looked for a doctoral thesis in which I could combine these two worlds, biophysics and machine learning. In Professor Andreas Schuppert’s research group, which was founded as part of the ASIC project in the SMITH Consortium, I then got this opportunity. At the time, it was about modelling a virtual patient to support AI-based methods. We wanted to bring the virtual patient and Deep Learning together to achieve early ARDS diagnosis.
You had just mentioned the virtual patient. What exactly is a virtual patient and how can it support the development of AI applications?
The virtual patient is a model that can describe certain states of patients using equations. For example, the cardiovascular or respiratory system can be represented with equations. If you bring these equations together, you can build a model that produces variables that can also be measured in the intensive care unit, e.g. oxygen saturation. Unknown characteristics of the patient are built into this model as parameters, for example the number of closed compartments (areas) in the lungs during ARDS. This models the pathophysiology of ARDS. The model should produce results that also exist in the real data. To do this, we try to find parameters for a virtual patient that make the difference between the measured data of the real patient and the results of the simulator as small as possible. We expect that these parameters will then be helpful in approximating the condition of the real patient. Instead of the measured data, we can then use the data from the model to follow the development of ARDS. This data can then be used to develop AI systems for the early diagnosis of ARDS.
Why is it useful to use data from the virtual patient model rather than the patient’s real data for AI-based prediction systems?
When we develop a model based on a particular dataset, the model learns the data distribution from just that dataset. If another data set that we feed into the model deviates from the training data set, then the performance of the predictive model becomes weaker. This is a problem especially in intensive care because the data from different hospitals are structured differently. There are many reasons for this, e.g. different admission strategies, treatment strategies and measured baseline values of the patients. This means that if we use only one hospital as a standard to develop a model, the model learns the characteristics of the condition in that hospital and not the general characteristics of the condition. This is where the virtual patient comes in.
In our study, we created a virtual patient for each of 1000 real patients with suspected ARDS from four hospitals. We automatically grouped the measured real data and the data from the virtual patients according to patients with similar characteristics. The result was that the original data from the hospitals sorted by hospital. When the data from the Virtual Patient was grouped, a separate group of patients diagnosed with ARDS could be found. Thus, the differences between the data from the different hospitals became smaller. This result shows that parameters from the virtual patient can help to increase the generalisability of AI models.
What role will artificial intelligence and machine learning play in intensive care medicine in the future?
I think AI and machine learning will not play a decisive but an important role in the future – especially in supporting doctors. In the research group, we looked at the critical conditions sepsis and ARDS, which are very dangerous for patients. The development of these conditions is a matter of hours or a day. If they are detected just a few hours earlier, so many patients can be saved. That’s where I see the potential of our models. They can be used like clinical decision support systems (CDSS) for early identification and prediction. These tools can help doctors focus attention on those patients who could develop sepsis within an hour, for example.
Secondly, medicine is currently evolving towards personalised medicine. I expect that AI methods will enable us to take targeted measures tailored to the individual patient at the right time. However, we must not forget that in the end it will always be the doctors who make the final decision about the necessary measures. Our AI tools will only serve to support decision-making, but I think they will never replace real doctors.
Please finish the following sentence: Research in the SMITH Consortium helps improve patient care because…
it brings together experts from different fields to produce tangible innovative results for the benefit of patients.